Ask the Expert
Pete Davis
Data scientist
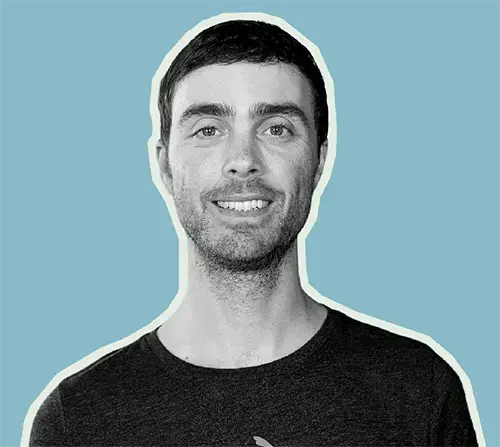
What challenges exist in identifying offshore oil and wind structures when developing fixed infrastructure models?
Creating a picture of what’s taking place beyond the horizon is far from easy. Our recent study analyzed 2 million gigabytes of satellite imagery to detect vessels and offshore infrastructure in coastal waters across six continents. This took nearly three years to do. One vital lesson we learned from all of this: identifying and classifying fixed offshore oil and wind structures requires a vastly different approach than what we use for vessel models. And this process is challenging for a number of reasons.Â
To develop our fixed infrastructure model, we first had to remove non-stationary objects, which were mostly vessels but can also include other floating objects like ice floes. We did this by using a time series of six-month synthetic aperture radar (SAR) median composites. Basically, we used SAR–a powerful remote sensing technology that works day and night in all types of weather–to eliminate background noise and paint a picture of the changing infrastructure at sea.
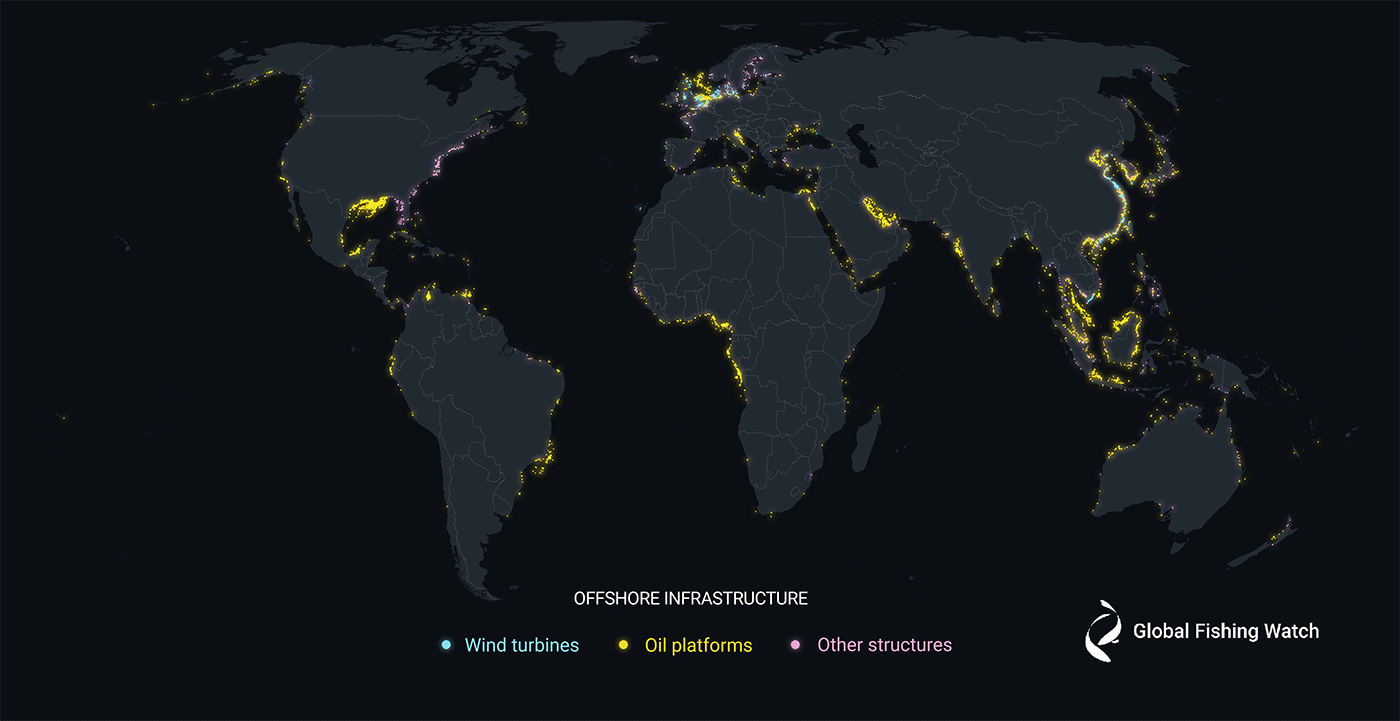
Global Map of Offshore Infrastructure Detections, 2017-2021
Two million gigabytes of satellite imagery were analyzed to detect offshore infrastructure in coastal waters across six continents where more than three-quarters of industrial activity is concentrated. â“’ 2023 Global Fishing Watch
Next, we worked on classifying detected infrastructure, which posed a new set of challenges. In contrast to the vessel detection models we’ve developed in the past, infrastructure classification incorporates high-resolution optical imagery from the Sentinel-2 satellite in addition to SAR from Sentinel-1. This provides another type of satellite imagery to help classify detections. To ensure that we got the most accurate dataset possible, we also put the classification results through many stages of extensive manual review by regional and domain experts.Â
Finally, we needed to develop a method to group detections over time to account for variance in an object’s location and label. For example, the same oil platform’s detected location may differ ever-so-slightly or it may be labeled differently between detections. To address this, we used a clustering method to group nearby objects and determine what to do when the labels didn’t match during different time periods. We then took the greatest average classification label to determine an object’s location and type.Â
The resulting first-of-its-kind dataset is helping us build a picture of what is taking place at sea, and we are proud to make it publicly available to those seeking more insight into our ocean and the activities taking place across it.